Dr. Watson Says: |
 |
...Just using multi-objective should increase your productivity and give you more insight into the problem... |
|
What Do You Say?
|
|
|
|
Let’s start with an example of multi-objective optimization. The map you see is all the towns and cities in Germany where your product is sold. Let’s assume you want to to determine how many warehouses you need so that you are within 75km of every customer.
This is a straightforward network optimization problem. However, you quickly realize that you don’t just want a single answer, you also want to know how much demand is covered within 75km by 5, 10, or 15 warehouses.
You could run many different scenarios to determine build out the trade-off curve.
Or, you could run a multi-objective run and get the full trade-off curve with a single run. The technical chart below shows the evolution of a multi-objective run. The box in the upper right is the starting point with a single solution. As you move to boxes 2 and 3, you see the optimization building the trade-off curve. The blue line is the best estimate of the trade-off curve. The green and red lines how how confident the solver is in that blue line. (When a red and green line touch, you know it has found a solution.) Box 4 shows a final answer—you now have a trade-off curve showing the percent of demand covered within 75km.
Previous Columns by
Dr. Watson |
|
|
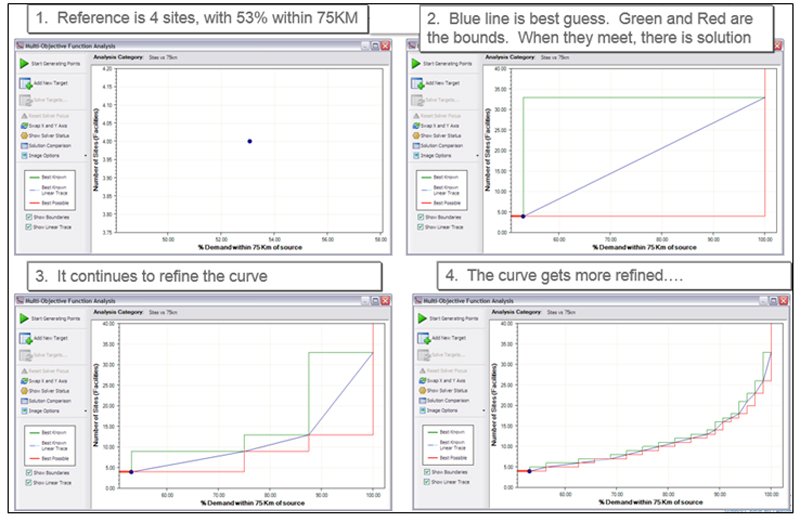
You can now quickly see that it will take 33 warehouses to cover all demand. But, you also see how much demand is covered by 5, 6, 7, … facilities.
This leads directly to our first best practice.
Best Practice #1: Use Multi-Objective Optimization
As you saw in the above example, you can quickly generate a complete trade-off curve with 1 run. This gives you quick insight into your solution. It also saves you time—this would have taken a long time to do one run at a time.
And, as you quickly realize, that you might want to also build this trade-off curve for 60km and 90km, and so on. Multi-objective makes this easy.
Just using multi-objective should increase your productivity and give you more insight into the problem.
Best Practice #2: Think Creatively About the Objectives
When I first teach optimization to a new audience, they almost always want to to consider multiple objectives. I think this a natural way to think about a problem. But, the use of optimization tools (including network optimization tools), have taught us to think of only one objective.
Once you realize the power of multiple objectives, you should think creatively about which ones to use. I’ve heard of clients that always force themselves to consider different objectives to gain new insights. The example above is an obvious scenario to run. But, some creative choices would be to trade-off manufacturing cost vs transportation cost or the cost of one division vs the cost of another division.
Best Practice #3: Use Multi-Objective Optimization for Objectives that Don’t Fit with Your Data
There are some costs that don’t naturally fit with other costs in your model. For example, a typical network optimization model will include a year’s worth of demand. So, your transportation costs will be an annual number. If you want to model capital investments (in new sites or equipment), you have to annualize this capital investment. But, instead of going through the pain of annualizing the cost, you could just set this as a second objective.
Another case is with customer pick-up costs. These costs don’t show up in the budget, but they are real to the overall supply chain. Instead of modeling them directly, you can set this as a different objective.
Best Practice #4: Use Multi-Objective Optimization When You are Stuck
Finally, multiple objectives come in handy when you are stuck. We worked on a problem where we needed to locate a new production line. This new line could do very fast change-overs. So, we not only wanted reduce transportation cost by putting this line in a good location, we also wanted this line to handle all the small batch-jobs in the system. We couldn’t figure out how to capture this until we realized that we could use set-up costs as 2nd objective. This allowed us to trade-off transportation costs vs set-up costs in the model.
|